T3 trains a common tactile representation from 13 sensors and 11 tasks
Tasks
- Masked auto-encoding (MAE)
- Material ID
- Object ID
- Contact area percentage
- 11 fabric properties (smoothness, friction, etc.)
- 3D delta pose between 2 frames
- 6D delta pose between 2 frames
- ... and more
T3 can be used as a tactile encoder for long-horizon manipulation.
T3 Architecture
Our model is a transformer-based architecture that takes in raw tactile sensor data and outputs a latent representation that is shared across all sensors and tasks. The model is trained with a multi-task learning objective that encourages the shared representation to be informative for all tasks.
Paper Code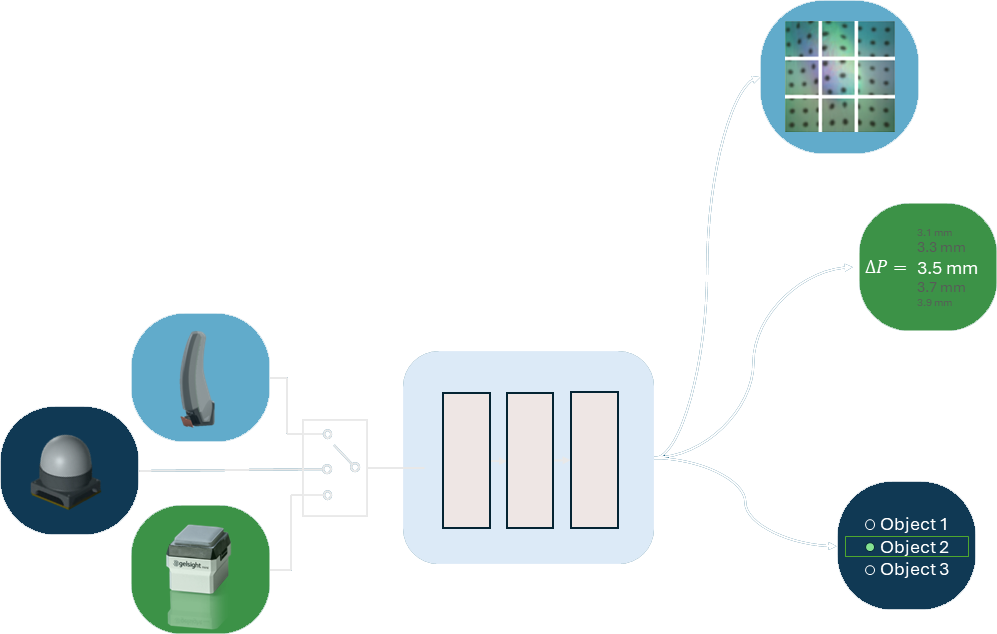
FoTa Dataset
The FoTa dataset is assembled by aggregating a few of the largest open-source tactile datasets, as well as additional "alignment" data collected by us in-house. It covers data collected 13 sensors and 11 tasks. FoTa is provided in a unified WebDataset format, which is pre-sharded for fast I/O.
Colab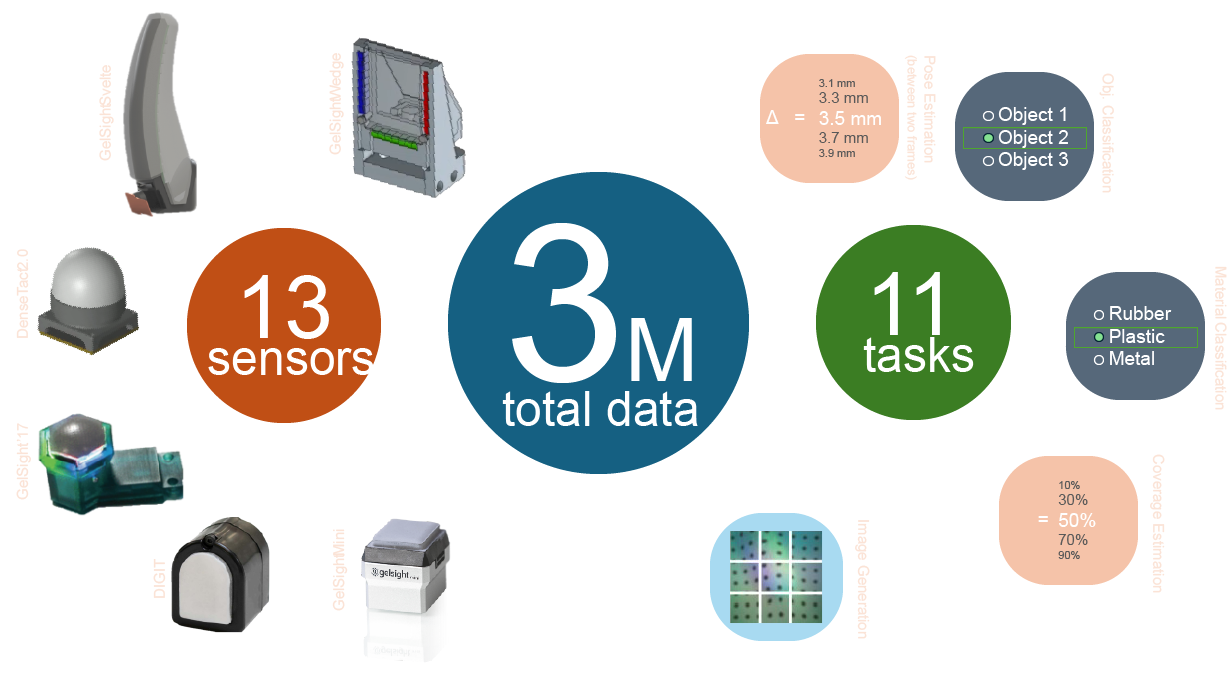
Abstract
This paper presents T3: Transferable Tactile Transformers, a framework for tactile representation learning that scales across multi-sensors and multi-tasks. T3 is designed to overcome the contemporary issue that camera-based tactile sensing is extremely heterogeneous, i.e. sensors are built into different form factors, and existing datasets were collected for disparate tasks. T3 captures the shared latent information across different sensor-task pairings by constructing a shared trunk transformer with sensor-specific encoders and task-specific decoders. The pre-training of T3 utilizes a novel Foundation Tactile (FoTa) dataset, which is aggregated from several open-sourced datasets and it contains over 3 million data points gathered from 13 sensors and 11 tasks. FoTa is the largest and most diverse dataset in tactile sensing to date and it is made publicly available in a unified format. Across various sensors and tasks, experiments show that T3 pre-trained with FoTa achieved zero-shot transferability in certain sensor-task pairings, can be further fine-tuned with small amounts of domain-specific data, and its performance scales with bigger network sizes. T3 is also effective as a tactile encoder for long horizon contact-rich manipulation. Results from sub-millimeter multi-pin electronics insertion tasks show that T3 achieved a task success rate 25% higher than that of policies trained with tactile encoders trained from scratch, or 53% higher than without tactile sensing.
More Details
Check more details about T3: Transferable Tactile Transformers from our paper or desktop website.
@misc{zhao2024transferable,
title={Transferable Tactile Transformers for Representation Learning Across Diverse Sensors and Tasks},
author={Jialiang Zhao and Yuxiang Ma and Lirui Wang and Edward H. Adelson},
year={2024},
eprint={2406.13640},
archivePrefix={arXiv},
}